As companies adopt advanced manufacturing technologies like IIoT, the volume of data threatens to overwhelm the bandwidth capacity of traditional cloud-based solutions. Many companies have moved much of their data to the edge to reduce these constraints and enable real-time, no-latency responses required for advanced automation.
Below, we’ll review this process in more detail, including how it works and why it’s critical in manufacturing today.
What is Edge Analytics?
Analyzing data at the edge is a method used to collect, process, and analyze data at some non-central point within a network—at the network's edge—rather than on the cloud or within another centralized system. This analysis usually occurs at or near a sensor, network switch, peripheral node, or some other connected device. The decentralized, local nature of analysis performed on an edge device has a benefit over more traditional big data methods in that it is much faster, leading to quicker, more accurate business intelligence while also lightening the load on the network.
In this model, the bulk of information is analyzed at the edge, and only the most relevant and critical information is then uploaded to the cloud for slower, deeper analysis. It is primarily the product of a growing number of IoT devices that require faster data analysis, sometimes in remote, unconnected areas, and can perform analysis at the edge and away from the cloud. In a way, it is a type of closed IoT data analysis ecosystem that has the ability to use a smart gateway to access the “outside world,” usually the cloud.
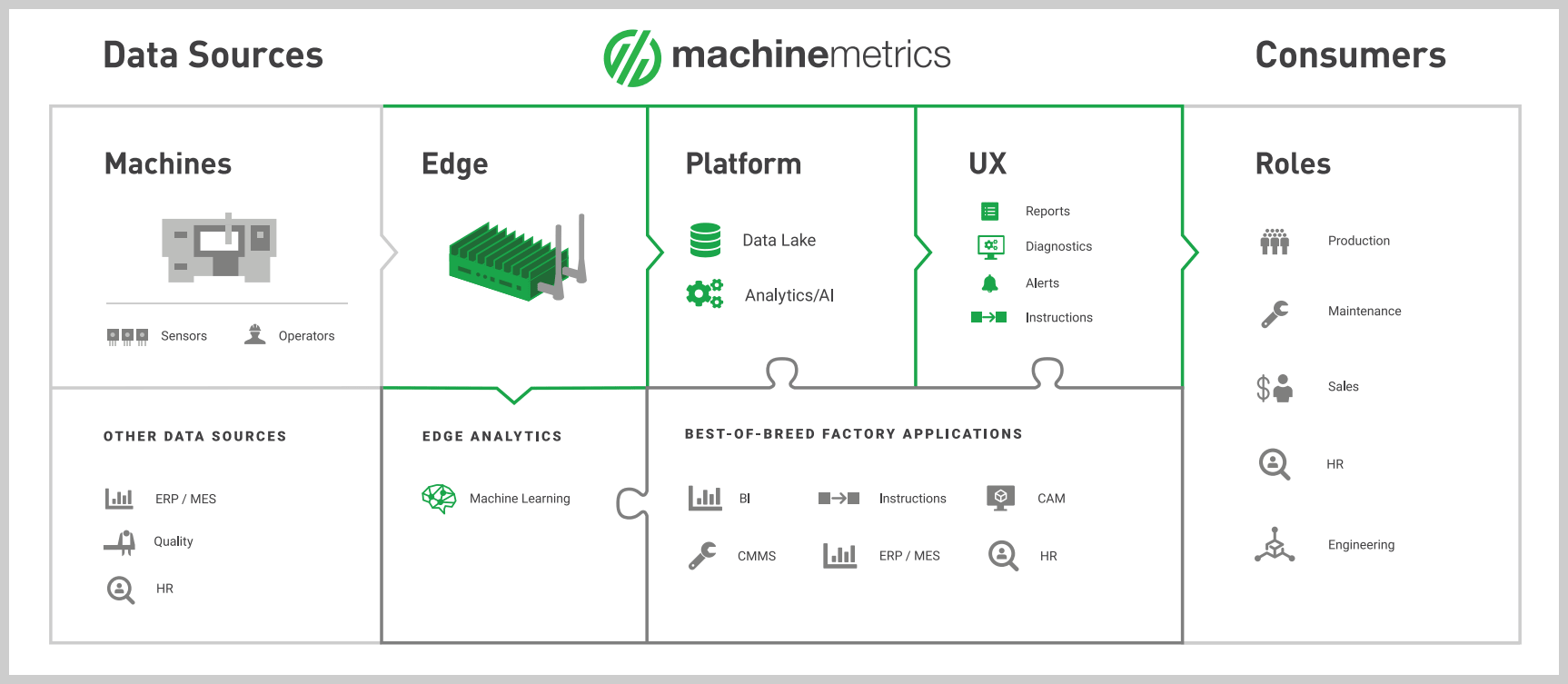
Periphery Data Assessment in the Internet of Things.
How Does it Work?
In a connected system using IoT or Industrial IoT, the amount of data gathered by sensors and other devices is enormous. The systems are deployed to efficiently use bandwidth and lower latency.
The device or edge computer uses three data types to determine action in these systems. It coordinates with the cloud-based platform to manage these data types and perform tasks.
It will first identify the type of data; if it requires no action, this data may be discarded as there are data points that have no use for system optimization. Other data is needed for record-keeping to be analyzed against current data to identify trends.
This analysis can be done at the edge, but it’s sent to the cloud for more complex analysis. Edge computing analytics filter, organize, and may even pre-process data before it’s sent to the cloud. Analyzing data at the edge requires action; the device will determine what action to take. These actions can include alarms, alerts, visualization data for HMIs, actuator triggering, machine shutdown, and more.
Edge Analytics vs. Edge Computing: What's the Difference?
Using the terms ‘edge computing’ and ‘edge analytics’ interchangeably would be easy. But there’s a difference. Edge computing refers to the infrastructure of edge devices and how they’re amassed at the edge to provide functionality.
Edge computing includes the edge devices embedded within the system components like sensors, actuators, routers, routing switches, and more. These devices may operate on an edge node or as part of an edge network. In edge computing, data flow management and traffic provide the infrastructure that enables components to work together within the system.
Data processing on the edge is the programming within edge devices that brings the data from multiple sources—aggregated, cleansed, pre-processed, and modeled—to determine the action needed. These actions may include trend analysis to be delivered to HMIs across a production floor, showing current and historical performance in a dynamic dashboard. When analyzing data, the system may determine the need for action, such as an alert triggering a cause-based machine shutdown.
Edge Analytics vs. Cloud Analytics
Both periphery data evaluation and cloud analytics perform data analysis to render insights or trigger action of connected devices. Here are their differences.
Analyzing at the edge is faster and requires significantly less bandwidth. It delivers low to no latency, which helps in environments like manufacturing where real-time data analysis and response are needed for precision operation or safety.
Cloud analytics is better for more in-depth analysis and massive data sets. Whereas analysis on an edge device is configured to address the needs of the system components and edge devices in its network. This configuration includes storage and processing; analytics capabilities are limited and dedicated to those functions.
Massive, raw data sets require cloud analysis with more processing capability. Cloud analytics also analyzes broader raw data within an enterprise and pulls in data from those resources as part of its data modeling.
For example, an edge computer used for analysis may be dedicated to machine monitoring, tool selection, or high-frequency data collection to trigger optimized operation and predictive maintenance for a set number of machines.
On the other hand, a cloud computing solution can analyze the insights from machine monitoring more deeply against results for the same machines in other facilities. It can also analyze it against finance data for complex costing and quoting programs irrelevant to the edge computer functions for managing specific machines.
Why is Edge Analytics Important Today?
Periphery data assessment is critical because it enables advanced manufacturing and smart factory proliferation to continue. Data creation quickly overwhelms network capacity as more embedded sensors and devices are added to equipment and manufacturing machines.
Rather than using central data analytics in the cloud, a decentralized system is required to allow IoT and other related technologies to continue their rapid growth. It removes massive amounts of traffic off networks and delivers insights and actions to connected devices near the point of data creation.
It also drives quick and accurate decision-making in advanced automation. This decision-making includes autonomous or semi-autonomous machine action alongside informed decision-making for operators, technicians, and managers using insights generated at the edge.
This analysis method drives advances in IIoT, advanced manufacturing, and automated cities. It continues to evolve manufacturing, driving increased efficiency and lower costs.
The Benefits of Edge Analytics
There are many benefits to edge analytics, including:
Speed
Commercial IoT applications and modern industrial operations require fast decision-making and real-time insights. These are necessary for the precision of automated functions and to improve safety. Analyzing data on the edge moves processing near the data to deliver the speed required.
Capacity
Technology is advancing within manufacturing automation, smart cities, autonomous machines, and other applications faster than cloud systems can manage in terms of bandwidth.
This dilemma risks ceding the advantages of real-time data to traffic jams and bandwidth congestion. This dilemma is resolved by moving processing where needed. It also preserves valuable bandwidth capacity for the most critical data analysis on the host platform.
Scalability
This type of system is also highly scalable. Edge computing can be added to an existing system, and edge networks can manage multiple edge computers across a network to keep data at or close to where the insights are needed.
System sizes vary wildly depending on the use case and industry, and this scalability allows small and enterprise-level deployment. It is also scalable based on the complexity and requirements of automation, from simple transactions to complex, highly-automated manufacturing functions.
Security
Security has been a lingering issue with cloud-based data. And while significant advances in cloud security have been made, analyzing on the edge presents substantial benefits. One is that because edge systems are deployed near the source of data, it has less distance to travel and, therefore, less chance of being breached.
The second advantage is that edge computing acts as a gatekeeper to provide an additional layer of security between data and the cloud through firewalls and other technology.
The Limitations of Edge Analytics
There are a few limitations to consider, such as that it may be overkill for small applications and use cases without speed. A second limitation is that it’s still a developing technology and not all edge devices and assets have analytics capabilities.
In addition, complexity may impede its utility. Edge devices are mission-specific and manage a clear set of equipment, machines, or devices. If data is needed for other analysis within the operation but not within the configuration of the edge computer, it can be lost.
IoT Edge Analytics Use Cases
IoT edge analytics are most beneficial to systems that require fast turnaround of data to guide functionality, IoT systems that collect massive swaths of data, and IoT devices that must function off-network for reasons of remote deployment or data security.
One prime example of inaction is the case of a military drone deployed in a remote location. Although technically, the drone can reach the outside world using satellite communications, data transfer speeds are slow—far too slow to effectively provide real-time feedback. Instead, using analysis at the edge provides near-instant feedback to ensure a safe mission, while critical data can still be securely uploaded afterward for further analysis.
Another example is its use in Industrial IoT. Machine health can be monitored in real time in a manufacturing environment leveraging the edge to detect anomalies that might indicate a failing tool and to monitor and provide feedback on production initiatives.
The speed of utilizing the edge for advanced analysis for IoT-enabled manufacturers is especially beneficial to prevent major safety incidents, reduce scrap part production, and to provide real-time statistics to production workers to better help everyone stay on track toward a goal. This is in comparison to cloud computing that, while it has its place in a manufacturer’s IoT strategy, would be too slow to offer the same levels of feedback and protection.
The IoT Edge Architecture
The IoT edge architecture consists of various devices that each play their role in an intelligent environment. They include:
Sensors
Sensors are the types of “things” people usually think of when they think of the Internet of Things. Sensors on the IoT measure variables such as temperature, light, location, moisture, and anything else that collects data from a physical object, such as a piece of manufacturing equipment.
Actuators
Actuators are what make changes within the IoT edge architecture. Think light switches, water valves, computer commands—anything that can make something else happen, such as turning on a light or distributing a software update.
Smart Devices
Many people are familiar with common smart devices like smartphones and tablets. Other examples of smart devices that live within IoT edge architecture include microcontrollers like Arduinos and single-board computers like the Raspberry Pi.
Smart Gateways
This is a critical component of IoT edge architecture. Smart gateways are like traditional IoT gateways, enabling data transfer between local devices and the cloud, but with additional “smart” features. These devices utilize common field protocols such as Bluetooth and Wi-Fi (among others) and can translate between these to cloud protocols like MQTT and HTTP. Industrial IoT Gateways can send local data to the cloud for storage and analysis as well as analyze local data for low-latency intelligence such as real-time anomaly detection.
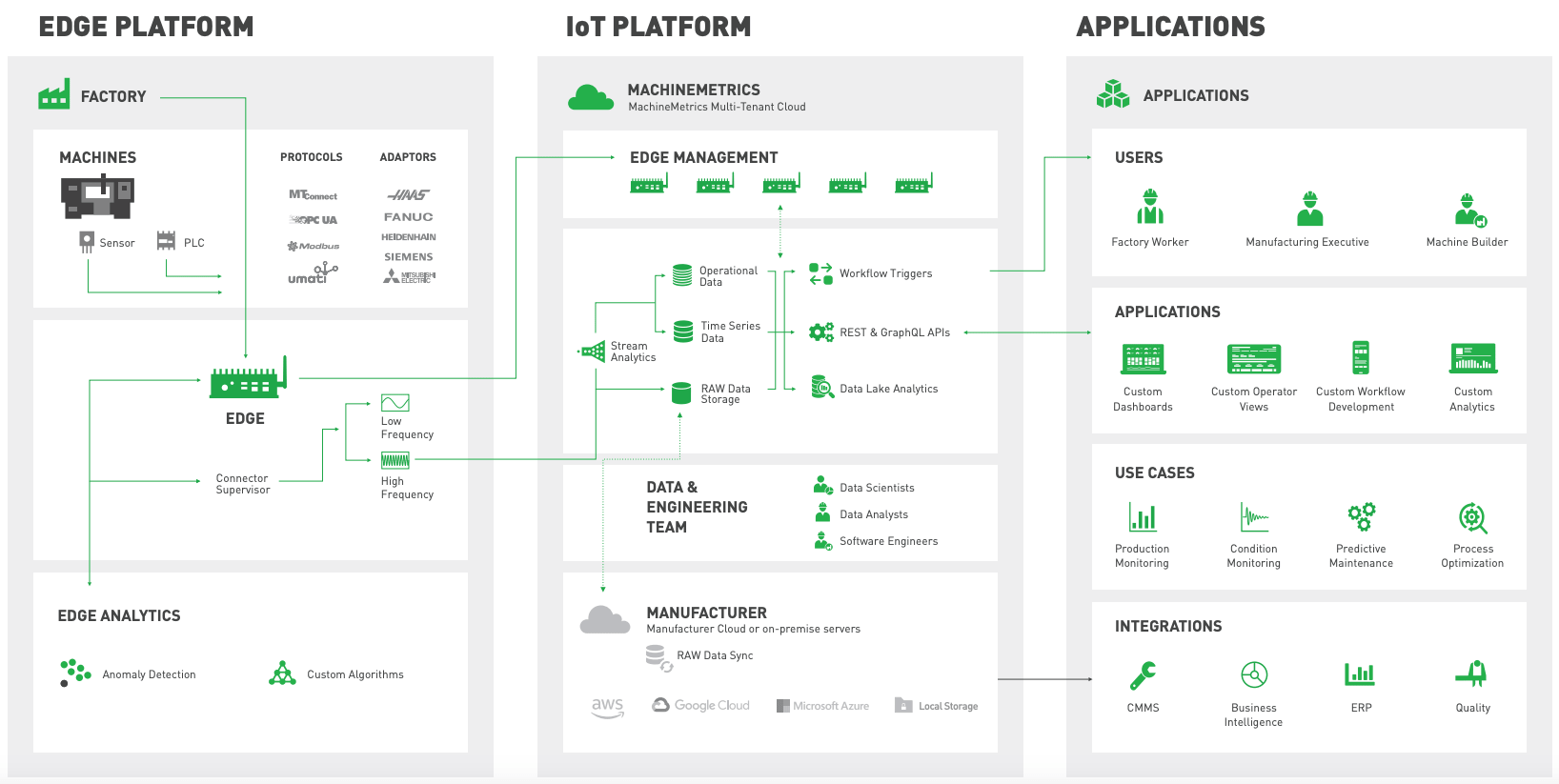
Edge Computing for IoT architecture.
Further Reading on Edge Analytics
For a more in-depth look at edge computing and analytics, including a deep-dive on edge platforms, edge devices, and the importance of the edge for manufacturing, read our eBook: "The Edge: A New Frontier for Manufacturing Analytics." Or explore the MachineMetrics Edge Platform.
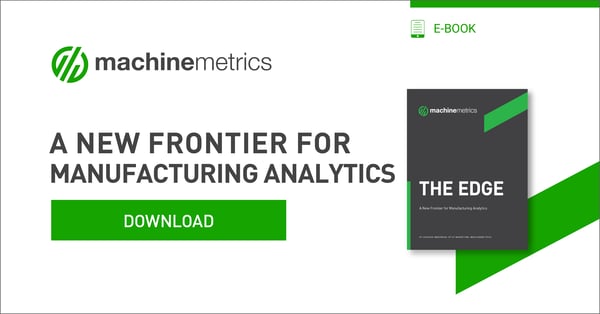
Comments